CR-708
Casey Spackman
College of Agricultural, Consumer and Environmental Sciences, New Mexico State University
Authors: Assistant Professor and Extension Range Management Specialist, College of Agricultural, Consumer, and Environmental Sciences (ACES), New Mexico State University. (Print Friendly PDF)
A drone flies over a cornfield at New Mexico State University. Photo by Josh Bachman & Derek Flodmand. NMSU, 2021.
Rangeland management is a complex and challenging endeavor. Effective management decisions necessitate thorough assessments of ecological conditions. Traditionally, these assessments rely on ground-based methods, which require extensive time and labor inputs. Given these limitations, particularly in large areas, it may be impractical to collect sufficient ground truth data to inform proper management decisions.
Novel technologies such as small, unmanned aircraft systems (sUAS) or drones are revolutionizing rangeland assessments. They offer faster and more feasible monitoring methods while also enhancing the accuracy of collected information. Drones capture high-resolution imagery of soil and vegetation characteristics, spanning large areas and providing a more comprehensive understanding of rangeland conditions. The information extracted from images can then be used to prioritize areas requiring strategic management directives, adjust existing management plans, and promote sustainable rangeland use.
Drones can modernize the way existing monitoring and management methods are employed on rangelands, but it critical to acknowledge the strengths and weaknesses of drone platforms before deployment. Essential information for effective drone use includes equipment selection, flight planning, assessment execution, and data analysis for generating prescription maps. Ultimately, understanding these concepts will enable range managers to become more efficient at monitoring and inform rangeland management decision-making.
Equipping the Drone
For accurate rangeland assessments, selecting the appropriate camera and sensors (payload) is crucial. Various types of payloads are available, each with its own advantage and disadvantage (Figure 1). Having a clear objective and knowing what the capabilities and limitations of a payload are can save time, money, and effort in the long run.
Figure 1. Various drone camera payloads for rangeland assessments: A) an RGB camera (DJI Zenmuse X7); B) a multispectral camera (Micasense RedEdge-P); C) a hyperspectral camera (Specim AFX series); D) a LiDAR sensor camera (Phoenix Scout series).*
A traditional RGB (red, green, blue) camera, also known as true-color camera, is the most common type used on drones. It captures visible light similar to the human eye, producing high-resolution colored images of the vegetation and landscape. These cameras are relatively affordable and user-friendly. However, they only provide information that is visible to the naked eye and therefore do not provide spectral (light not visible to the human eye) or in-depth information for assessing vegetation cover, condition, or composition.
Multispectral cameras offer another option for drone-based assessments. They capture images in several spectral light bands beyond the visible range, such as the red edge or near-infrared. These bands can be interpreted as normalized indices, providing valuable information about plant health, photosynthesis rates, growth characteristics, biomass production, and species composition. By analyzing the reflectance of different wavelengths of light, multispectral cameras enable the detection of various landscape characteristics, including vegetation conditions, cover, and density. While multispectral cameras are more expensive than RGB cameras, they offer greater detail and insight about the landscape.
Hyperspectral cameras represent a more advanced technology compared to multispectral cameras, capturing images across hundreds of narrow spectral light bands, thus offering continuous signatures. This provides even more detailed information about the landscape, including the chemical composition of plant leaves and soil. While hyperspectral cameras are significantly more expensive than other options, they provide highly detailed and exponentially useful information.
Alternatively, LiDAR (Light Detection and Ranging) scanners can also be used on rangeland drones, providing detailed information about the structure and density of the landscape. LiDAR sensors emit pulsed laser light to analyze reflectance time and create highly detailed three-dimensional terrain and vegetation maps. This data can be useful for assessing habitat quality, estimation of vegetation structure, distribution and mass, and creating high-resolution topographical maps.
Finally, thermal or infrared cameras are used to detect heat signatures in the environment. They enable the assessment of soil and vegetation characteristics, including moisture content, temperature distribution, and surface conditions. While they can be used as a standalone payload, thermal cameras are often integrated into other payloads, such as RGB and spectral cameras.
Regardless of the payload, the drone should be equipped with GPS and telemetry systems to track its position and flight data. This ensures the operator knows the drone’s location during flight and facilitates easier data processing post-flight. Ultimately, the choice of payload is determined by factors such as cost, flight objectives, and desired level of information detail.
Planning the Flight
Flight planning is a critical step before utilizing drones for rangeland assessments. Plans should consider the topography and mapping area, determining the flight path and restrictions, setting altitude and imagery overlap for adequate data resolution, evaluating weather conditions, and implementing safety protocols to mitigate flight risks or hazards.
The defined boundaries or area for assessment are limited by the drone’s capability and the terrain. Rules set by the Federal Aviation Administration (FAA) restricts drone flights to visual line of site (VLOS), despite the capability of the drone to fly further. In steeper terrain, VLOS may be limited by the desired mapping area. Additionally, battery life or fuel capacity may impose constraints on flight duration for mapping. In some cases, dividing the area into smaller sections may be necessary to ensure adequate data is collected in a single flight. Flights may also be divided up to allow for refueling or battery replacement.
Figure 2. Drone flight path in a parallel survey grid with 75 percent side overlap.
Once the topography and drone limitations are known, the flight path and altitude can be determined. Flight paths typically follow sequential parallel paths (Figure 2). To cover the entire area of interest and generate sufficient outputs for processing, images or paths should have a minimum overlap of 75 percent. However, overlap requirements may vary based on recommendations of the data processing software and assessment objectives. Furthermore, flight altitude will be influenced by camera quality and desired level of image detail. For instance, higher altitudes with lower overlap offer broader coverage but less detail, while lower altitudes with greater overlap will cover smaller areas with greater detail (Figure 3).
Figure 3. Classified orthomosaic from a drone flight using ArcGis Pro software.
The flight plan should also consider weather conditions, flight restrictions, and safety protocols. Know the aircraft’s limitations and always check the weather before flying, as conditions can change rapidly. Additionally, airspace restrictions may be present, requiring permissions. It is important to obtain any necessary permits or authorizations before commencing a flight. The FAA offers preflight planning services known as B4UFLY, provided by four private companies—Airspace Link, AutoPylot, Avision, and UASidekick—accessible through desktop and mobile applications.
Flight safety is paramount. A comprehensive flight plan should include safety protocols that address emergencies and potential obstacles, such as buildings, trees, or power lines. The drone should only be operated by a certified operator who adheres to FAA rules and regulations. For detailed drone rules and regulations, visit the FAA website: https://www.faa.gov/uas
Conducting the Flight
The quality and accuracy of the collected data depend on the proper execution of the flight. Before conducting the flight, physical examination of the drone and its components should be done to ensure proper function. The drone itself should be inspected for any loose parts or defects, and all batteries should be fully charged and free of defects.
Additionally, the payload should be securely fastened and properly configured according to pre-flight specifications. The flight should be conducted in accordance with the flight plan but may be adjusted if any issues or hazards arise. During the flight, maintaining the appropriate altitude and speed is essential, and the camera or sensor should trigger at designated intervals. The pilot in command (PIC) must maintain VLOS at all times, avoiding direct flight over people, vehicles, or other obstacles. At times, the flight plan may need adjustment based on weather conditions, unexpected obstacles, or other aircrafts within close proximity. If any factors increase the risk of equipment damage or harm to individuals, the flight should be postponed or cancelled.
Throughout the flight, the PIC should monitor telemetry data to ensure that the drone is functioning properly, adhering to the planned flight path, and data is accurately collected. Any anomalies or issues should be promptly addressed to prevent data loss or equipment failure.
Once the flight concludes, the data should be downloaded from the drone and securely stored. Subsequently, the data can be processed and analyzed using appropriate software and appropriate techniques for the specific assessment objectives. In summary, conducting a successful flight requires careful planning, attention to detail, and adherence to safety guidelines and regulations.
Processing the Data
To extract meaningful information from rangeland drone assessments, the collected data must undergo processing using specialized computer programs. Some examples include Pix4D, which produces drone imagery tiles, and ArcGIS software, typically used for calculating normalized vegetation indices and prescription maps. The selected program must be capable of handling large data sets from various types of cameras or sensors. Higher-resolution imagery and larger flight areas will require more computing power. The data can exist in various formats, such as images or point clouds, depending on the payload. Processing techniques are tailored to the specific data collected and the assessment goals.
Initially, the raw data must be converted into an analyzable format. This may involve converting image data into an orthomosaic or tiles—a contiguous map of overlapping images. Increased overlap between images makes combining images easier and removes distortion caused by drone movement or camera lens characteristics. A seamless image enhances the assessment of ground characteristics like vegetation cover, density, and bare soil content.
Once the data is in a usable format, a variety of geostatistical techniques can be used.
- Object-based image analysis (OBIA):
- Grouping pixels within the orthomosaic into smaller, more manageable clusters based on characteristics such as color and texture. Clusters can then be classified based on their attributes (Figure 3).
- Spectral Analysis:
- The process of analyzing different wavelengths of light to extract meaningful information such as vegetation indices.
- Normalized Difference Vegetation Index (NDVI) provides information on plant health, type, and leaf mass, determined by the amount of light reflected and absorbed; higher reflectance values indicating denser and healthier vegetation (Figure 4).
- Enhanced Vegetation Index (EVI) provides the same information as NDVI but accounts for atmospheric background noise and high reflectance values from dense vegetation.
- Soil Adjusted Vegetation Index (SAVI) is opposite of EVI, accounts for high reflectance values of soil, and is generally used where sparse vegetation is found.
- Point cloud:
- A processing technique that uses individual pixels and data points to generate three-dimensional models of terrain, structures, and vegetation quantities (Figure 5). Each point in a cloud represents a discrete location in three-dimensional space and may include information such as color, intensity, or reflectance values.
- LIDAR sensors capture spatial coordinates and attributes of individual laser data points to create a highly detailed three-dimensional point cloud.
- Advancements in computer programs can now process RGB imagery (photogrammetric) into point clouds based on multiple overlapping images and predicted individual pixel location.
- Point clouds can be used to create detailed digital representations of real-world objects or environments. While highly detailed maps generated from point clouds offer advantages, the large size and complexity of processing may present challenges.
Figure 4. NDVI resolution comparison at different flight altitudes (above ground level; AGL).
Figure 5. Photogrammetric three-dimensional point-cloud from a drone flight using Pix4D software.
In conclusion, data processing is a critical step in using drones on rangelands and is highly dependent upon the quality of data collected during flights. The processed data provides valuable insights into vegetation condition and density, erosion, and invasive woody species, empowering land managers to make informed land management decisions.
Analyzing the Results
Once the data has been processed and analyzed, prescription maps can be produced and used. This stage of application involves interpreting the data to identify patterns, trends, and areas of concern, and using this information to formulate recommendations for management strategies. It is essential to thoroughly review the results to ensure the quality and accuracy of the data. This may entail checking for errors, anomalies, and inconsistencies in the data, and verifying that it accurately represents the assessed area. Drone imagery serves as a valuable tool designed to complement, not to replace, traditional on-the-ground methods and streamline assessments. In cases where anomalies are detected, on-the-ground assessments may be required to validate the image’s accuracy.
One method of data analysis is to compare current maps with previous assessments. This facilitates tracking landscape changes and conditions over time, as well as identifying areas of concern. Visualization through maps, charts, and other mediums aids in informing management decisions. For instance, a significant increase in bare ground since the last assessment may indicate issues with grazing, drought, or other factors requiring immediate attention. Increased levels of bare soil can lead to erosion, soil degradation, reduced water quality, and habitat loss. Recognizing these risk areas enables land managers to proactively implement mitigation measures such as reseeding, erosion control structures, or grazing exclusion to prevent further degradation.
Another approach to leverage prescription maps is by comparing areas that differ from the rest. Sites exhibiting low vegetation abundance may indicate continuous heavy livestock use or soil nutrient deficiencies. Conversely, areas displaying high vegetation abundance may indicate areas of heightened wildfire risk, invasive species expansion, or under-utilized livestock areas. Identifying these areas empowers land managers to prioritize management and allocate resources more effectively. Any management strategy addressing concerns must be grounded in science and entail a comprehensive understanding of the data’s strengths and limitations. Decisions should also reflect the specific goals and priorities of the management agency or organization, which may include strategies for restoration, invasive species control, or livestock management strategies. Furthermore, integrating drone imagery with satellite-based remote sensing products extends the scale of rangeland monitoring with minimal compromise of informational detail or accuracy.
Conclusion
Drones offer a cost-effective and time-efficient approach to assess landscape conditions and make informed management decisions on rangelands. They transform the scale of rangeland monitoring, allowing for comprehensive assessments without compromising informational detail. Integrating satellite-based remote sensing products further extends monitoring capabilities to ranch, watershed, and regional scales. This novel technology has the potential to revolutionize rangeland monitoring, assessment, and management practices. Drones enable the collection of highly accurate, fine-scale data in a more efficient manner compared to traditional ground-based or remote sensing techniques. However, effective use requires a thorough understanding of key concepts. This includes careful design of flight plans, selection of appropriate equipment based on management objectives, and access to specialized computer programs for data processing. As these technologies advance, drone usage will become more streamlined and user friendly. However, drones are intended to complement rather than replace on-the-ground assessments, offering significant time and cost savings. Ultimately, the data collected by drones enhances our understanding of rangeland conditions, inform management decisions, and promotes better land management practices.
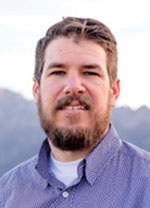
Casey Spackman is an Assistant Professor and Extension Range Management Specialist at New Mexico State University. He earned his Ph.D. at Utah State University. His Extension efforts aim to assist producers, land managers, and agency personnel in monitoring and developing management objectives that maintain or improve natural resource health and sustainability.
To find more resources for your business, home, or family, visit the College of Agricultural, Consumer and Environmental Sciences on the World Wide Web at pubs.nmsu.edu.
Contents of publications may be freely reproduced, with an appropriate citation, for educational purposes. All other rights reserved. For permission to use publications for other purposes, contact pubs@nmsu.edu or the authors listed on the publication.
Brand names appearing in publications are for product identification purposes only. No endorsement is intended, nor is criticism implied of similar products not mentioned. Persons using such products assume responsibility for their use in accordance with current label directions of the manufacturer.
*The images included in this publication are used for illustrative purposes and their specific sources are not always identified. Every effort has been made to credit sources where possible. If you recognize any images and can provide information on their origin or require proper attribution, please contact us at pubs@nmsu.edu
New Mexico State University is an equal opportunity/affirmative action employer and educator. NMSU and the U.S. Department of Agriculture cooperating.
September 2024 Las Cruces, NM